PROBLEM AND MOTIVATION
Semantic Imagery Mapping (SIM) is an interface for thematic and compositional analysis of large image collections. Developed as part of the research project Borrowing Algorithmic Epistemologies, led by Prof. Elias Bitencourt, SIM moves beyond traditional approaches that often rely solely on grouping images by superficial characteristics or descriptive labeling provided by computer vision APIs.
SIM stands out by combining various computer vision models to create visualizations that uncover not just formal similarities but also the underlying contexts and themes within image collections.
Designed to meet the needs of researchers and designers working with extensive image datasets, SIM provides a machine learning interface to explore aesthetic and semantic trends, visual imaginaries, and the social and cultural dynamics of image circulation.
Beyond Google API Labels and Formal Similarities
DEVELOPMENT CONTEXT
Semantic Imagery Mapping (SIM) is a core part of the Borrowing Algorithimic Epistemologies project, coordinated by Prof. Elias Bitencourt. This project investigates how the epistemological logics of algorithms—the ways computational systems perceive, organize, and interpret the world—can be adapted as analytical tools for studying social, cultural, and communicational phenomena.
SIM not only applies computational logic to identify patterns in large image datasets but also enables investigations into how these logics shape visibility and communication processes in digital platforms.
By adapting methods of visual and semantic analysis, SIM fosters a critical understanding of the sociotechnical dynamics that influence the circulation of narratives and imaginaries in digital environments. It provides tools to map and interpret these phenomena in detail, revealing how visual practices and cultures are structured and transformed in these spaces.
Viewing Images Through Algorithmic Lenses
HOW IT WORKS
Pattern Identification and Image Clustering
Images are preprocessed, and their features are extracted using a combination of computer vision models. This allows the identification of patterns based on both compositional and thematic similarities, connecting images through narratives and visual contexts. The resulting clusters represent shared visual themes or concepts.
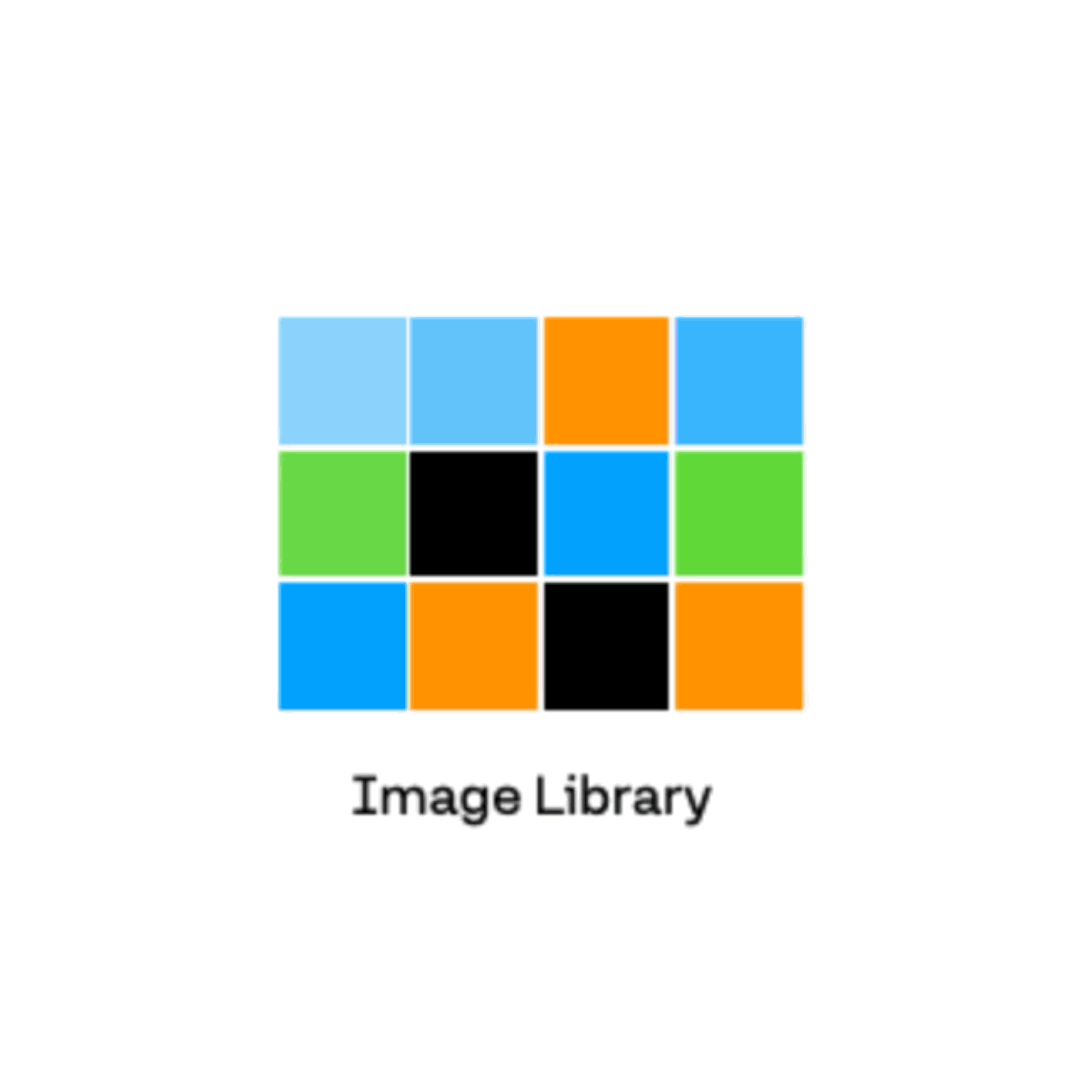
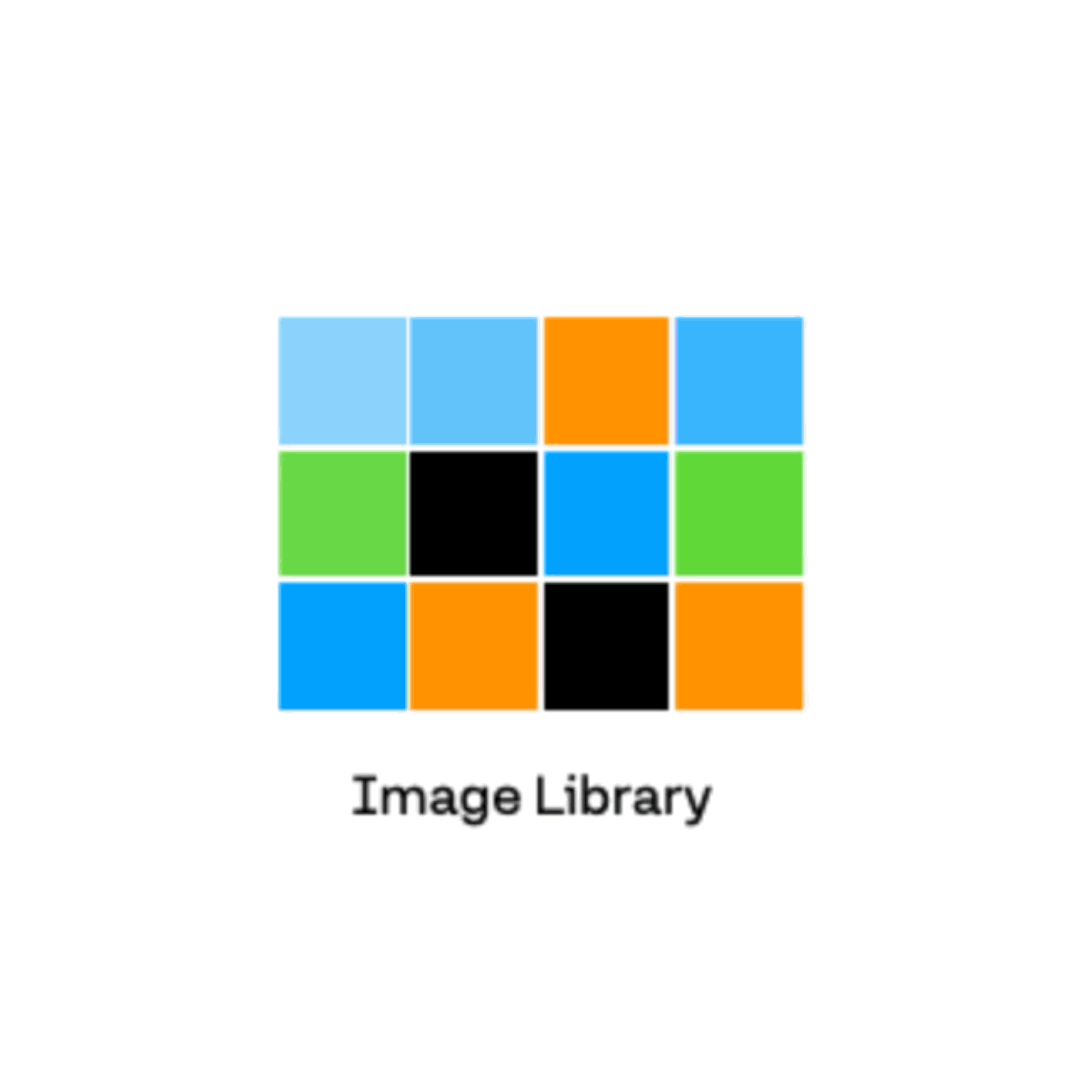
Image Wall
Each cluster is assigned a distinct color, with images within clusters organized by similarity level. Clusters themselves are arranged based on visual proximity, creating an image wall layout that provides an intuitive overview of dominant patterns.
In the example, an image wall showcases 2,688 book covers organized into 37 literary genres identified by SIM.
Contextualized Automatic Labeling
When textual metadata such as captions or hashtags are available, SIM generates automatic labels for clusters based on this information. This approach focuses on identifying contextual topics associated with the use and circulation of images, rather than describing them in isolation.
Cluster Validation and Refinement
SIM includes features to identify thematic relationships between clusters, suggesting possible manual regroupings to improve data cohesion and interpretation.
Additionally, SIM generates detailed reports with quantitative metrics and indicators that describe clusters and assess the consistency and quality of groupings, supporting analysis and decision-making throughout the data exploration process.
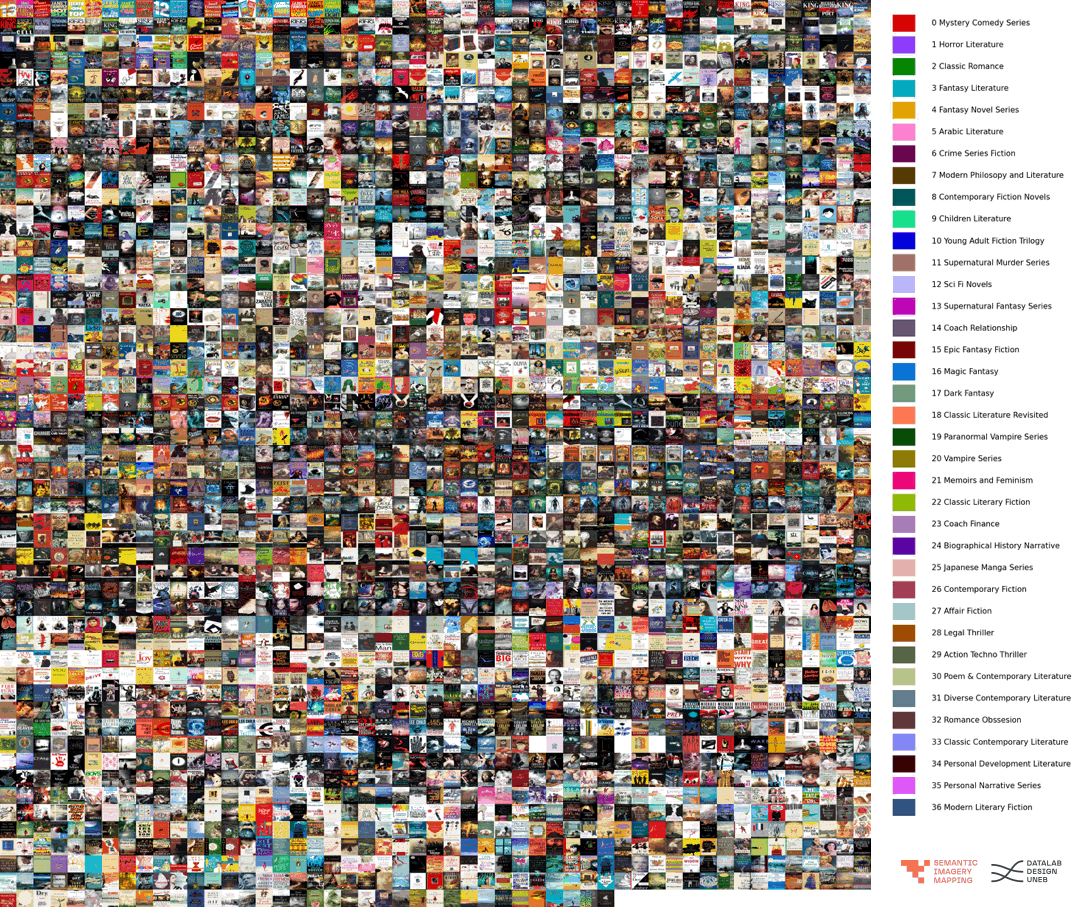
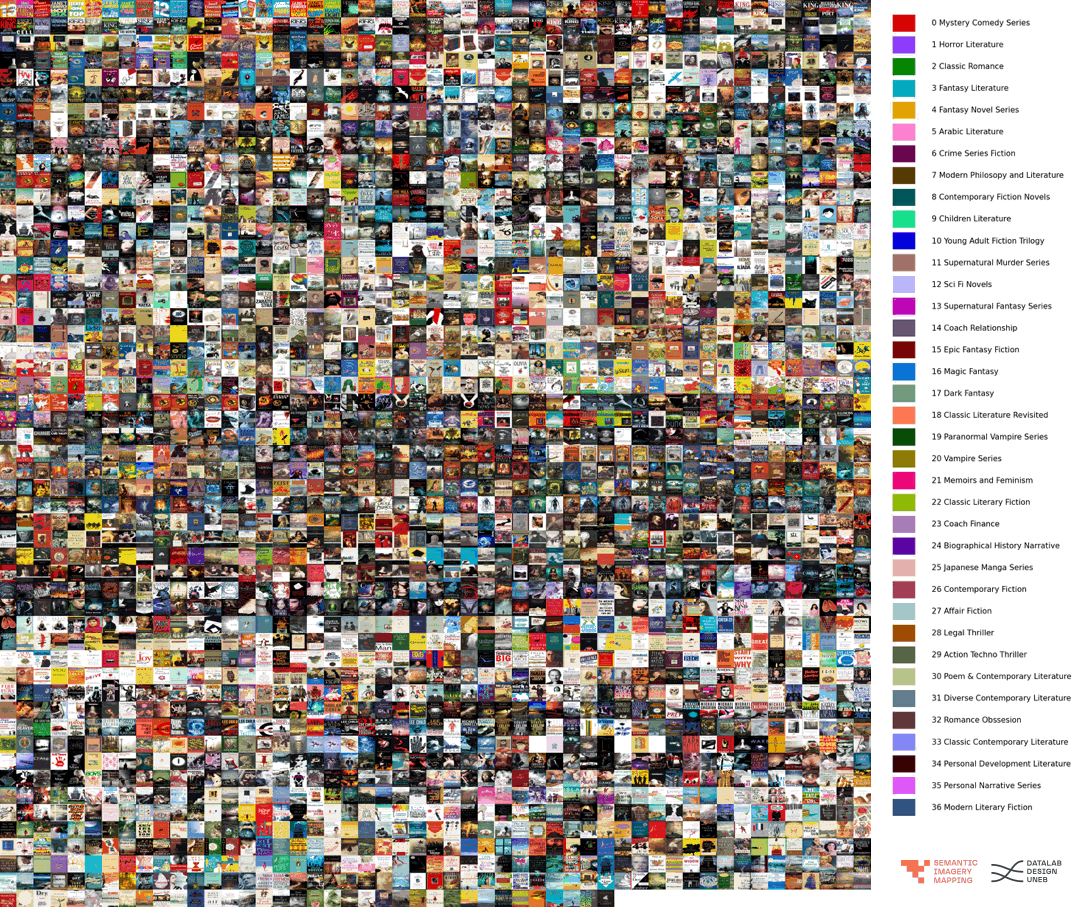
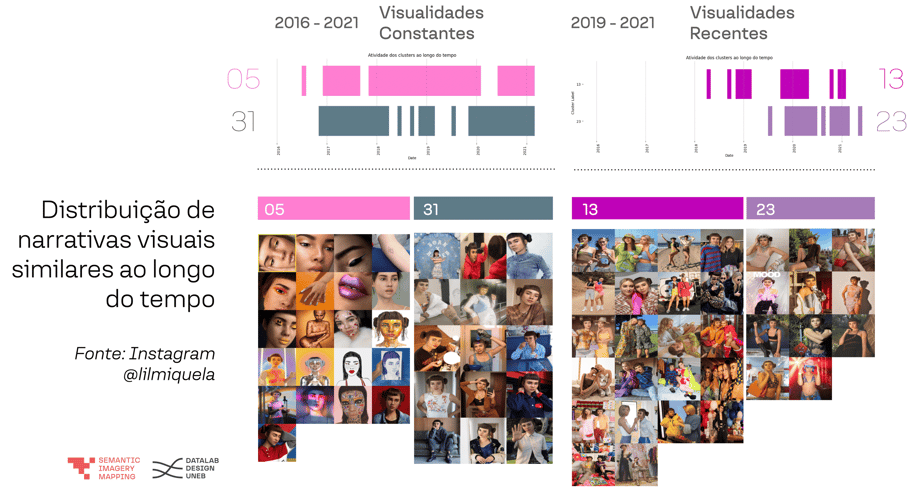
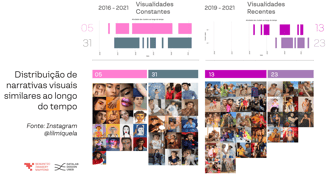
Visualização temporal
The tool also enables analysis of how visual patterns evolve over time, distinguishing between persistent narratives and specific trends. This functionality is useful for mapping narrative cycles and thematic shifts.
One example features an image wall of 1,080 Instagram posts by CGI influencer @Lilmiquela, with horizontal lines indicating the distribution of clusters over time, spanning 2016 to 2021.
Zooming in, we see that clusters 05 and 31 appear consistently between 2016 and 2021, while clusters 13 and 23 only emerge after 2019. This visualization helps analyze which themes, compositions, and narrative styles define the influencer as a whole and which are tied to specific moments in her trajectory.
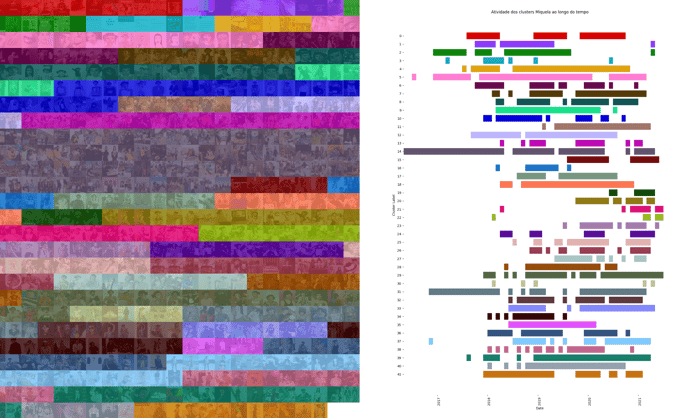
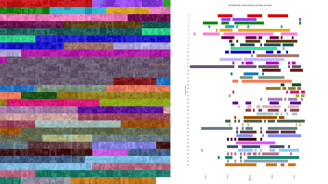
Thematic Maps
Clusters are displayed in thematic maps that visualize relationships between the semantic and compositional patterns in the corpus. These maps make it easier to identify connections, contrasts, and relevance among groups.
Closer clusters reflect higher similarity, while distant ones highlight significant differences. Central clusters represent broadly connected themes, whereas peripheral clusters emphasize specific, distinctive elements.
For example, in the map of 2,688 book covers, biographies share visual references with financial self-help books. Contemporary fiction emerges as the genre with the most varied layouts, while Arabic literature and horror are isolated at the edges, indicating highly specific visual patterns.
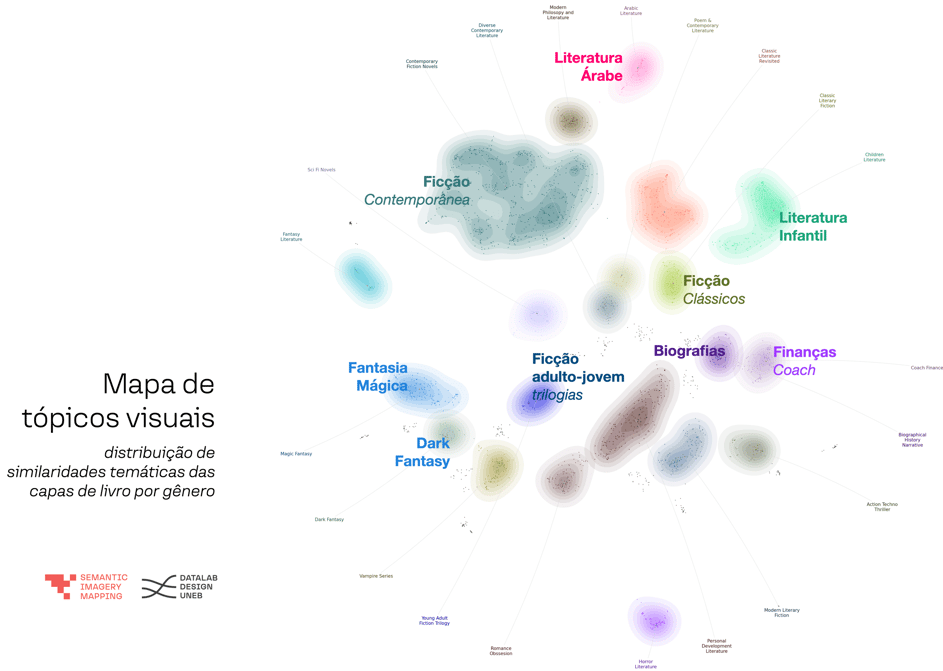
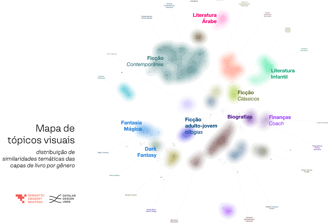
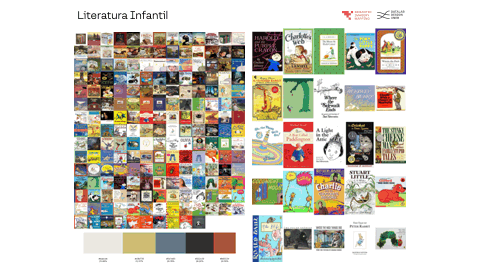
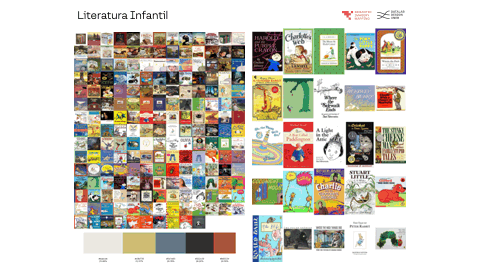
Finding Representative Images
SIM synthesizes the most representative images from each cluster, highlighting those that best capture the visual and thematic characteristics of each group. This feature facilitates quick and detailed interpretation of the clusters.
POTENTIAL APPLICATIONS
Mapping Visual Narratives: Identify thematic, stylistic, and narrative patterns in large image datasets, providing insights into how visual concepts emerge and connect in different contexts.
Visual Archive Curation: Assist in organizing and classifying image collections, highlighting thematic and compositional similarities to facilitate cataloging and analysis.
Analysis of Stylistic Patterns: Map aesthetic trends in artistic, editorial, or commercial collections, identifying recurring themes and innovations in visual styles.
Temporal Exploration of Narratives: Track the evolution of visual patterns over time, distinguishing consistent narratives from seasonal or contextual variations.
Comparative Analysis of Visual Datasets: Explore similarities and differences across datasets, series, or collections, highlighting how different groups represent themes or address visual issues.
Support for Creative Curation: Provide insights for selecting images or building visual narratives in editorial projects, exhibitions, advertising campaigns, or audiovisual productions.
Data Visualization and Design: Create graphical representations that synthesize visual similarity patterns in a corpus, translating large image datasets into actionable analyses.
Mapping Visual Imaginaries and Consumption Patterns: Identify dominant visual imaginaries associated with brands, campaigns, or social events, offering insights into the reception and circulation of visual content.
Visual Influence Analysis: Evaluate how visual patterns connect with specific audiences, exploring how certain styles or themes resonate within different communities.
Imaginaries Trends Narratives Curation
AVAILABILITY AND ACCESS
Using Semantic Imagery Mapping in Research
Currently, Semantic Imagery Mapping is under development and limited to ongoing research within the laboratory. It is not yet available for general use or broad distribution.
Collaborations may be considered for specific projects aligned with the lab's research agenda and team availability. Proposals for collaborations or partnerships will be reviewed based on their potential contribution to ongoing investigations.
If you are interested in exploring collaboration or investment opportunities, please contact the group leader for preliminary discussions: eliasbitencourt@gmail.com.